Computational photography in smartphones photography brings resolution
Of course, taking that image of an astronaut on the surface of the moon from earth with a phone, is a joke. But it somewhat explains the exponential improvement.
From recognizing the subject to improving it, all of this is being possible due to the use of computation in photography.
What is computational photography?
Computational photography involves the use of specialized software algorithms aiming to improve the quality of the images.
For a smartphone, it’s like having a mini photographer inside, doing all the adjustments for you while you just have to tap the shutter button.
The reason why computational photography is so beneficial for smartphones:
Google’s head design engineer stated
The lens and sensor are pretty much at the limitation controlled by physics and computational photography is the way to grow now in performance.
Not every smartphone user is a photographer:
Unlike professional photographers, all smartphone users doesn’t necessarily have the knowledge of how to construct a good image, how to manage different parameters like the shutter speed, the ISO and all that technical stuff. It’s great when you just have to tap the shutter to take a great image.
All this is possible with the use of various specialized software algorithms for different specific purpose. It does all the adjustments and calculations for you.
Small smartphone sensors capture less information:
Smartphone camera sensors are limited by space. A larger sensor increases the focal length making it hard to fit inside a phone. But on the other hand, smaller sensors captures less information about the image. Often the images appear to be noisy and also with less detail due to less data input (light).
Thankfully, computational photography is here to help reduce the noise and artifacts generated by tiny photosites. These software processing methods becomes very helpful for devices with high resolution sensors that has even smaller photosites or pixels.
Sub-par lenses causes chromatic aberration
The lenses are often made of lower quality materials than that of a dedicated camera. It often causes artifacts like chromatic aberration and lens flare on the image. A bright source of light in a darker background presents awful lens flares.
These kind of artifacts can be reduced with the help of specific software processing algorithms.
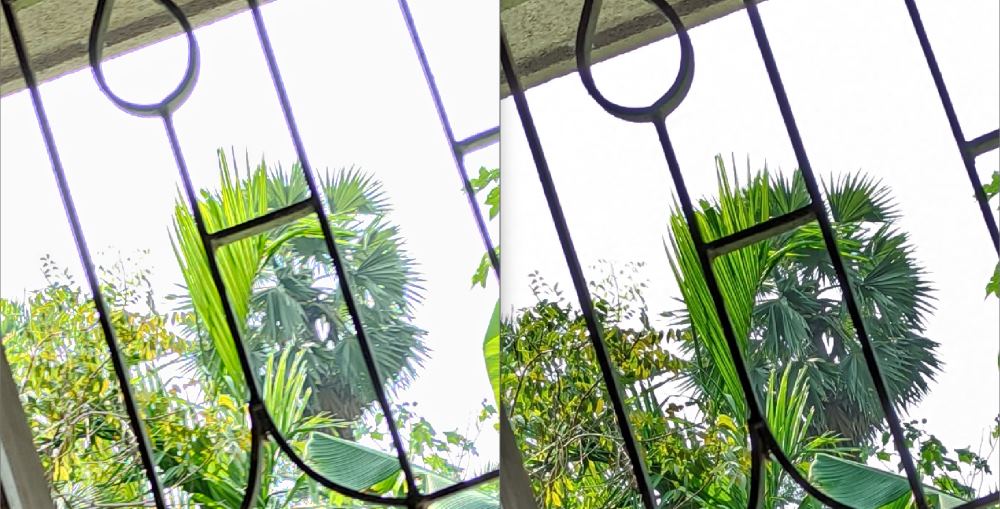
Its hard to believe that both the images are created using the same lenses. However it has been made possible by google camera’s clever software processing. This is one of the example of how far computational photography can go in terms of removing artifacts and improving the image overall.
We took the images above from a entry level Xiaomi device using it’s stock camera and the Google camera (modified version of Google Pixel’s system camera app). The right one is from Google camera, which is known for it’s computational imaging capabilities.
It’s interesting to see how google camera’s computational processing has been able to completely remove the chromatic aberrations on the rods.
By using clever software processing, smartphones gets rid of many of these. It would be very difficult to solve these issues on the hardware level. Computational processing is actually a life saver for the smartphones in this regard.
How computational photography improves image quality?
Image stacking
Stacking involves capturing multiple images of a subject with variations in parameters such as exposure, focus, or position, and merging them into a single image to create a result that cannot be achieved with a single shot.
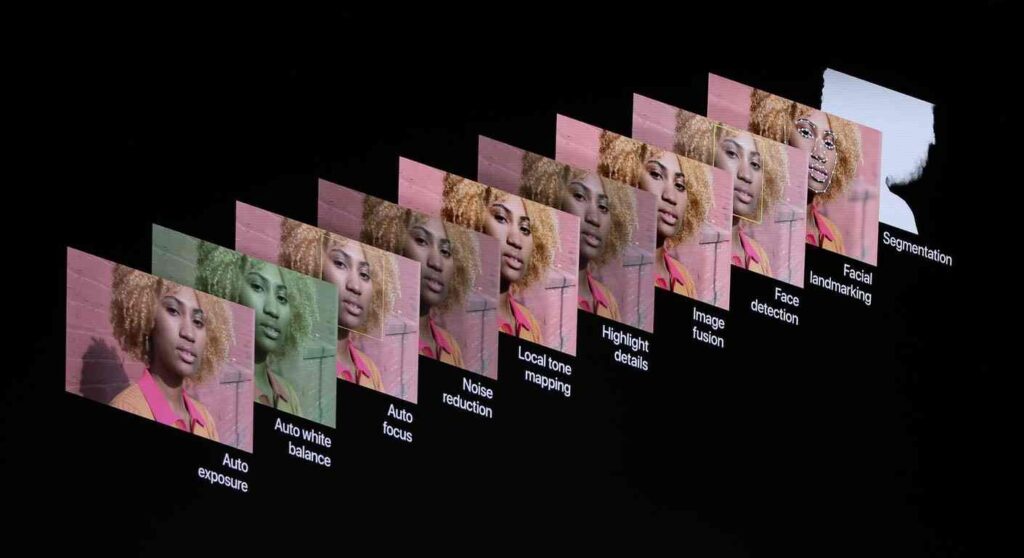
This technique forms the basis of over 90% of the computational photography methods employed in smartphones.
HDR
Notice how the HDR shot has details better on the bottom of the leaf. It’s also the fruit of stacking images of different exposure levels together.
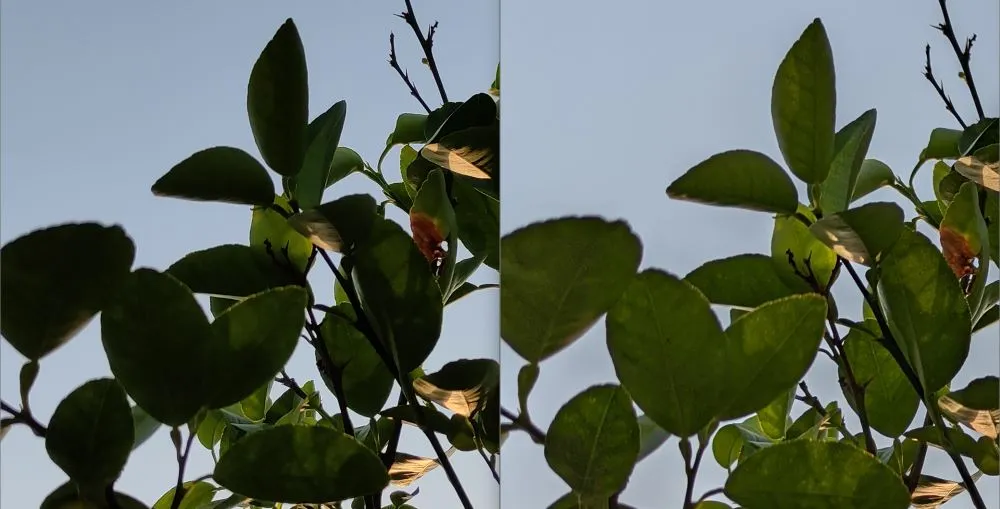
The dynamic range of the mobile sensors are usually not enough to capture high contrast scenes like bright sky and a darker foreground correctly in a single frame. Either the sky gets blown out or the foreground appears black.
But smartphones have computing power. It captures multiple frames with different exposure levels. It uses specialized machine learning algorithms to detect the over and under exposed parts of the scene by analyzing the pixel values. Now it merges properly exposed parts from those images to construct an image that properly exposes the whole frame.
Google Pixel’s HDR+
In low light conditions, exposure time of the long exposure shots gets long enough to cause motion blur to the images due to our hand movement. Either the exposure time has to be reduced or else a blurry image.
To address this issue, Google took a slight different approach in the nexus devices. The technology is called HDR+
HDR+ takes a burst shot with very short exposure times, obviously they appear under exposed. Then it chooses the sharpest of the shots and aligns the others with it algorithmically. By averaging the pixel values from all the shots, HDR+ reduces the noise in the final image. After this step, the shadows are lifted to create a brighter image that has improved detail and reduced noise.
And all this seems to work. I see HDR+ performing better than the conventional HDR processing almost every time when testing different devices.
Night mode/ Night sight
Here is another application of image stacking. It takes multiple frames of relatively shorter exposure to avoid motion blur due to hand movement. Then the frames are aligned and merged together to get a bright as well as crisp shot.
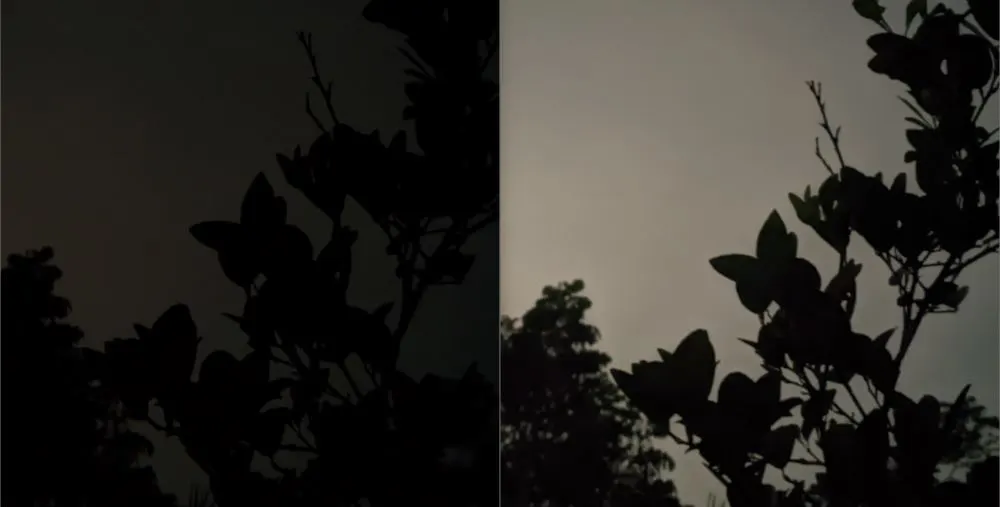
The images above show how stacking multiple images can be helpful for low light conditions. Using a longer exposure would require
In a continuous long exposure shot, the device must stay still for the whole time, making tripods necessary. Computational photography is what enables us to take hand held images in low light. For a professional camera, you will need to use a tripod for this purpose. Yes, software processing is this much helpful in some specific cases.
Portrait mode
Smartphones don’t have larger lenses to create a natural bokeh effect. However, they have the processing power as well as the required software to differentiate the background from the subject and artificially add a blur effect to it. The edge detection isn’t perfect all the time, but it’s getting better with improving machine learning algorithms.
Noise reduction
Noise is one of the biggest problem in smartphone images and computational processing is a life saver in this regard. It can differentiate the noise (unwanted variation in color and luminosity of the pixels) from useful information.
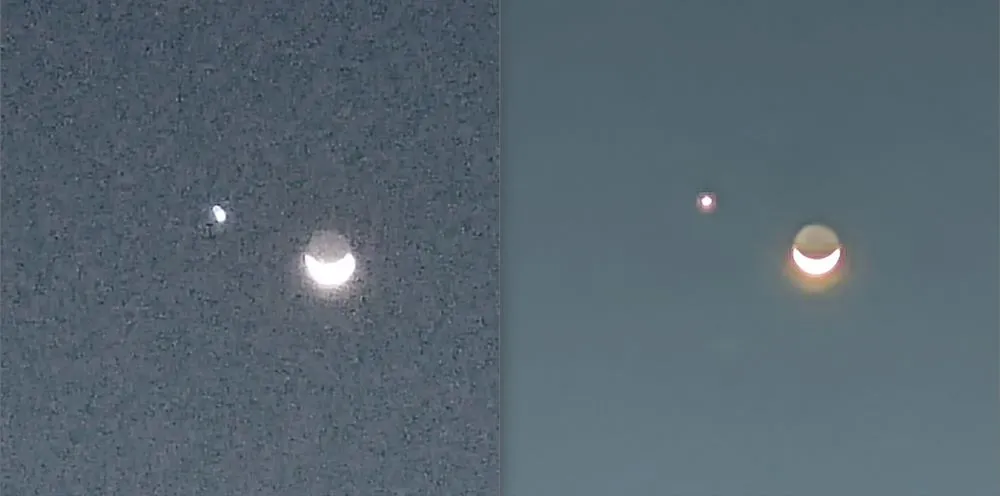
Both of the images are created by the same hardware, the difference you see is due to the use of sophisticated noise reduction algorithms of Google Camera.
The noise can be corrected using computation, as reflected in the second image. The values of adjacent pixels as well as those from different frames are analyzed to estimate a more accurate value, resulting in reduced noise and a cleaner final image.
Taking shots handheld
From the above images, you can observe that the stock camera sample suffers from motion blur. In fact, I took multiple shots from both cameras handheld, and most of the samples from the stock camera had motion blur, whereas not a single one from the Google Camera was blurry. We can see Google’s aligning and stacking in action!
These images demonstrate the contribution of computation in photography
Samsung’s Space zoom
The space zoom feature relies heavily on software enhancements. After taking multiple shots, besides aligning and averaging it also uses machine learning to recognize different subjects and improve them accordingly.
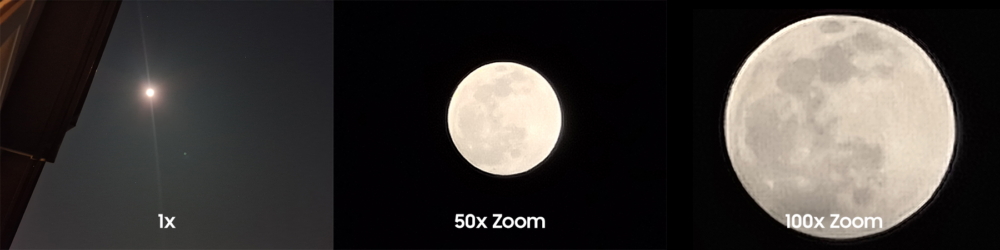
In specific cases like taking a picture of the moon, it recognizes the moon and improves detail on it based on the information about the moon it already has. Even if clouds are covering the textures, it can still take an image where the textures are present to make it look good.
Features like this won’t ever be possible in a smartphone just using the optics. Computational photography is contributing a lot in smartphones lately, which is a good thing.
Some other eye catchy features
Pixel’s face unblur feature
When there is a moving face with respect to the camera, it uses both the main and ultrawide lens. The main camera is operated at a shutter speed according to the lighting condition ( that can introduce motion blur to the face) and the ultrawide is operated at a faster shutter speed to avoid motion blur to the face.
After taking both the images, if the face is blurry, it merges the face from the ultrawide’s image and enhances that using machine learning. Finally you get an image that’s correctly exposed and yet the face doesn’t look blurry.
This feature available on newer Google Pixel devices, including the Pixel 6 & 7 series. Pixel 7 series devices can also unblur a face from an image already taken using the Google Photos app.
Pixel’s super resolution zoom
This feature was introduced with the Pixel 3 series. It retains detail in images while zooming digitally. Though it isn’t a complete replacements for dedicated optical and telephoto zoom lenses, it retains much more detail compared to a digital zoom without the software enhancements.
It involves a technology called sub-pixel level offset that captures multiple frames of an image at slightly different positions, where the movement or offset is smaller than the size of a single pixel in the camera sensor. This means that the camera captures the same scene from slightly different angles or positions, with each frame containing slightly different information about the image. After analyzing and aligning the frames the system is able to create an image with better detail.
The sub-pixel level offset technique is achieved using complex algorithms that take into account the characteristics of the camera sensor, the optics of the lens, and the movement of the camera during image capture. The algorithms also applies a deblurring technique to reduce any blur caused by camera movement or hand shake during the capture.
Is it always helpful?
Our smartphone is basically a pocket computer, so using its computational power to overcome the limitations of its compact form factor (i.e., smaller optical equipment) is a great idea.
However, the quality improvement depends upon the use of algorithms. While flagships excel in this aspect, more affordable devices usually don’t have access to those advanced algorithms. As a result, we often see over-processed and unrealistic images that honestly look bad. Computational photography is great, but only with it’s proper implementation.