Pixel binning in smartphones
Pixel binning is a method of merging data from multiple pixels of the camera sensors in order to obtain more accurate information from the pixels.
Pixels are divided into smaller pieces to play with megapixel numbers, and then they have to be merged together using additional processing power to maintain quality.
You may have seen marketing materials claiming that by merging a number of pixels, you get a much larger pixel that increases light capture, resulting in a better image. While it’s true that binning simulates a larger ‘super-pixel’, they rarely mention how much they have shrunk the sub-pixels in the first place.
For context, the iPhone 13 Pro’s 12MP sensor has a pixel size of 1.9µm, whereas it is only 0.6µm in the 200MP sensor of the Galaxy S23 Ultra. While clever software processing can influence the final output, it’s always better to start with better information, which isn’t the case for these binning users.
How much of an improvement pixel binning brings?
The improvement in images isn’t quite due to the binning process; rather, it’s due to the fact that phones aren’t able to process the images at full resolution. Pixel binning reduces the resolution, allowing the device to run its image processing algorithms, which actually improves the images.
I was trying to see how much of an improvement pixel binning can bring in low light conditions and here’s what I found.
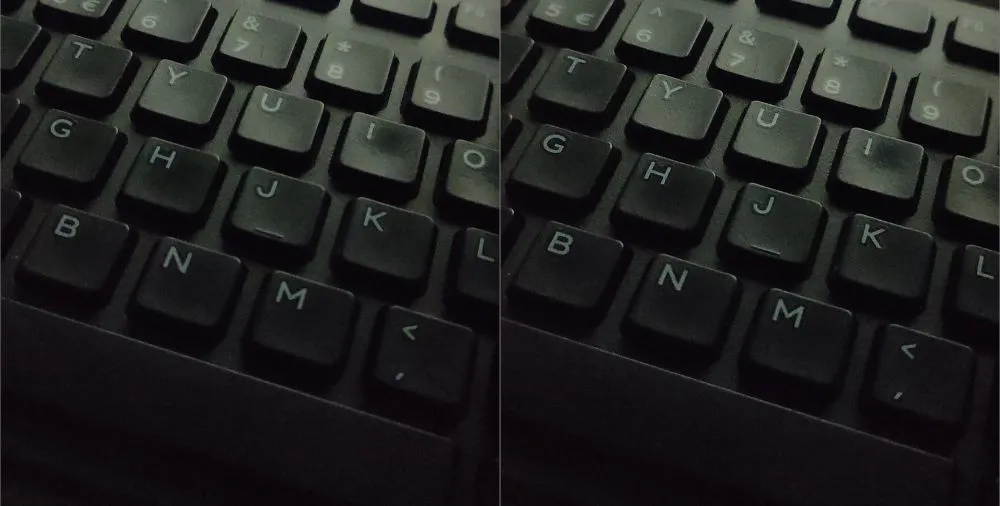
One is at 16MP and the other is at 64MP, but you can barely tell which is which. It’s a budget device that doesn’t have access to sophisticated computational processing techniques to enhance the images in its camera application in the first place.
Pixel binning is overrated for its actual impact. Its primary function is to enable the device to utilize software processing techniques in order to enhance images. However, not every device has access to such advanced algorithms, and pixel binning fails to match the expectations here.
(the left one was the full resolution shot)
Pixel binning don’t increase the amount of light capture
Well, the amount of light capture depends on the sensor size. For a specific sensor size, the light capture will be the same for all sensors regardless of their resolution.
Pixel binning merges multiple pixel and virtualizes a bigger “superpixel”. This “superpixel” having the total area of all the pixels merged, it takes the collected amount of light of the smaller sub-pixels. This way the superpixel gets more light information than the smaller subpixels, but the total amount of light absorption in the sensor remains the same.
Pixel binning vs fewer but Larger pixels: Which one is actually better?
Binning improves the signal-to-noise ratio at the expense of resolution, but it still cannot match the SNR (Signal-to-Noise Ratio) of standard low-resolution sensors. The CMOS binning process involves reading out all the pixels and then merging them outside the sensor using software processing. However, this readout process introduces read noise for all the pixels, which cannot be completely eliminated during merging.
Physical pixels of a given size typically exhibit better SNR than simulated ‘superpixels’ and also impose a lesser workload.
For example, a standard 12MP sensor with larger pixels, despite having lower resolution, often delivers superior native signal-to-noise ratio. Additionally, providing better information to the ISP (Image Signal Processor) enables enhanced computational photography capabilities.
The standard resolution sensors also put less workload on the device, which consumes less power, while the larger pixels provide better information.
How Pixel Binning fails to match the signal-to-noise ratio of a 12mp sensor?
- Tetracell or quad pixel. (4-1 binning) improves the SNR by two times compared to that of the smaller subpixels
- Nonacell i.e. 9-1 binning improves the SNR by 3 times compared to its subpixels
- Tetra2 binning (16-1binning) improves the SNR by 4 times than the the subpixels
However, the standard 12MP sensor still maintains a higher signal-to-noise ratio than any of the above. Want to know how? Let’s break it down. Assume a 12MP sensor with the same surface area as a 48MP sensor (in reality, they are not much different).
The pixel area of the 12MP sensor is 4 times bigger than that of the subpixels in the 48MP sensor. Now, the pixel area is proportional to its SNR value, so the subpixels in the 48MP sensor have 4 times lower SNR value than the larger pixels of the 12MP sensor.
The merged ‘superpixels’ of the 48MP sensor get a 2 times improvement in the SNR value. But the 12MP sensor still has the edge of 2 times more signal-to-noise ratio than the high-resolution sensor.
As we have already discussed, read noise is introduced to all the pixels before binning. Averaging the pixel values doesn’t fully eliminate the read noise.
Do pixel binning reduce resolution or image size?
Yes, it reduces the resolution by one-fourth or one-ninth or one-sixteenth, based on the number of pixels merged together.
Final take
We divide the pixels and then merge the data from these pieces, which requires additional calculations and power consumption. The ironic part is that, despite all the sacrifices, binning ultimately results in a relatively lower signal-to-noise ratio (SNR) than a physically large pixel (as we have discussed above), which isn’t worth the compromises made.
In return we get the flexibility to take high resolution images in well-lit conditions. However, your megapixel requirement will vary depending upon your specific use cases.